-
이미지 처리 부분은 전체 공개되지 않았다.
-
추론, 인식, 설명, 코딩 능력이 대단하다.
-
회계, 법률, 의학 등 전문 분야에서도 뛰어나다.
-
특히 수학을 매우 잘 한다. 물 완벽하지는 않다.
-
비디오, 오디오 멀티모달 관련 부분 이야기는 없다.
-
공개 모델에 대한 이야기를 하면 디스코드에서 차단당한다.
When will GPT-4 be released? Immediately, but through a waitlist. How can I access GPT-4? There are a few ways to access GPT-4: • Join the waitlist. • Subscribe to ChatGPT Plus. Plus users will have access to GPT-4 through the dropdown menu. • Contribute to the OpenAI Evals. If your pull request is accepted, you will be taken off the waitlist. Is an API for GPT-4 available? Yes. Once you get access to the model through the waitlist, you will have access to the API. How much will it cost to use GPT-4? • $0.03 per 1000 tokens for prompt tokens. • $0.06 for completion tokens for the 8K token engine. The 32K engine is double the price per token. How is GPT-4 different from GPT-3? GPT-4 has a similar architecture to GPT-3 but was trained with much more computer power. What new features and capabilities does GPT-4 have? GPT-4 shows improvements across the board and performs better in various tasks. Notably, it exhibits higher performance in professional tasks, showcasing novel capabilities. How can I access the image recognition feature of GPT-4? This feature is nt yet available to the public. Is this a research preview of GPT-4? No.
Join Greg Brockman, President and Co-Founder of OpenAI, at 1 pm PT for a developer demo showcasing GPT-4 and some of its capabilities/limitations. Join the conversation on Discord here: discord.gg/openai(https://discord.gg/openai). We'll be taking audience input from #gpt4-demo-suggestions. - https://www.youtube.com/live/outcGtbnMuQ?feature=share
# GPT-4의 답변
1. 저는 AI 텍스트 기반 모델로서, 직접 영상을 생성할 수는 없습니다. 하지만 제가 제공하는 정보를 바탕으로 영상 생성 프로그램이나 다른 도구를 사용해 영상을 만들 수는 있습니다. OpenAI는 이미지 생성에 적합한 다른 AI 모델, 예를 들면 DALL-E와 같은 것들을 개발했습니다. 이러한 모델은 텍스트 기술을 사용해 이미지를 생성할 수 있습니다. 영상 생성에 관심이 있으시다면, AI 기반의 이미지 또는 비디오 생성 도구를 찾아보시는 것이 좋습니다.
2. 죄송하지만, 저는 텍스트 기반의 AI 모델이기 때문에 직접 이미지를 생성하거나 제공할 수 없습니다. 그러나 OpenAI의 DALL-E와 같은 이미지 생성 모델이 이미지를 생성하는 데 사용될 수 있습니다. 혹시 이미지 검색 엔진을 사용하여 검색하시거나, 인터넷에서 고양이 이미지를 찾아보시는 건 어떨까요? 다양한 고양이 사진을 찾아볼 수 있을 것입니다.
3. 현재 기준으로 가장 최신의 AI 모델은 GPT-3입니다. GPT-4에 대한 정보는 제한적이며, GPT-4의 능력과 한계에 대해 자세히 설명하기는 어렵습니다. 그러나 일반적으로 AI 언어 모델의 한계와 발전에 대해 설명해드릴 수 있습니다.
4. 현재 GPT-3에 대한 정보만 가지고 있기 때문에 GPT-4에 대한 구체적인 정보를 제공할 수 없습니다. 그러나 일반적으로 언어 모델과 이미지 처리에 대해 설명할 수 있습니다. GPT-3와 같은 대부분의 언어 모델은 텍스트 기반 입력에 최적화되어 있습니다. 그러나 멀티모달 AI 모델은 텍스트와 이미지를 동시에 처리할 수 있는 능력을 가질 수 있습니다. 이러한 멀티모달 모델은 이미지 및 텍스트 데이터를 모두 이해하고, 이를 바탕으로 추론하고, 적절한 출력을 생성할 수 있습니다. 예를 들어, OpenAI의 CLIP 모델은 텍스트와 이미지를 모두 처리할 수 있는 멀티모달 AI 모델입니다. 이러한 모델을 통해 이미지와 텍스트 간의 관계를 분석하고, 이미지에 대한 설명을 생성하거나, 텍스트 설명에 맞는 이미지를 찾는 등의 작업을 수행할 수 있습니다. GPT-4가 있다면, 이러한 멀티모달 능력을 가질 가능성이 있습니다. 하지만 GPT-4에 대한 구체적인 정보가 없으므로, 현재로서는 확실한 답변을 드리기 어렵습니다.
# 공식 페이지
GPT-4는 더 넓은 일반지식과 문제 해결 능력 덕분에 어려운 문제를 더 정확하게 해결할 수 있다.
GPT-4는 그 어느 때보다 더 창의적이고 협력적이다. 노래 작곡, 각본 작성, 또는 사용자의 작문 스타일을 배우는 등 창의적이고 기술적인 작문 작업에 대해 사용자와 함께 생성, 편집 및 반복할 수 있다.
GPT-4는 25,000단어 이상의 텍스트를 처리할 수 있어 긴 형식의 콘텐츠 제작, 확장된 대화, 문서 검색 및 분석과 같은 사용 사례를 지원합니다. GPT-4는 이미지를 입력으로 받아들이고 캡션, 분류 및 분석을 생성할 수 있습니다. GPT-4가 첨단 추리능력에서 ChatGPT를 넘어섰다. GPT-4는 수험생 중 대략적인 백분위수에서 높은 점수를 받아 ChatGPT를 능가한다.
균일 봉 시험
우리는 GPT-4를 더 안전하고 정렬하도록 만드는 데 6개월을 보냈습니다. GPT-4는 불허 콘텐츠 요청에 대한 응답 가능성이 82% 낮고, 내부 평가에서 GPT-3.5보다 사실적 대응 가능성이 40% 더 높다.
인간의 피드백을 통한 훈련
우리는 GPT-4의 행동을 개선하기 위해 ChatGPT 사용자가 제출한 피드백을 포함한 더 많은 인간 피드백을 통합했습니다. 우리는 또한 50명 이상의 전문가들과 함께 AI 안전과 보안 등 도메인의 조기 피드백을 위해 일했습니다.
실전 사용의 지속적인 개선
우리는 GPT-4의 안전 연구 및 감시 시스템에 이전 모델을 실제 사용하는 교훈을 적용했습니다. ChatGPT와 마찬가지로, 우리는 더 많은 사람들이 그것을 사용함에 따라 GPT-4를 정기적으로 업데이트하고 개선할 것입니다.
GPT-4지원 안전성 연구
GPT-4의 고급 추론과 지시에 따른 능력은 우리의 안전 작업을 신속하게 진행했습니다. 우리는 GPT-4를 사용하여 교육, 평가 및 모니터링에 걸쳐 모델 미세 튜닝과 분류기에 대한 반복을 위한 교육 데이터를 만드는 데 도움이 되었습니다.
GPT-4는 대형 멀티모달 모델(이미지와 텍스트 입력을 받아들이고, 텍스트 출력을 방출한다)으로 많은 현실 시나리오에서 인간보다 능력이 떨어지는 반면 다양한 전문적이고 학문적인 기준에서 인간 수준의 성과를 보여준다.
2023년 3월 14일 이항
기존의 많은 ML 벤치마크가 영어로 작성되어 있습니다. 다른 언어의 초기 역량 감각을 얻기 위해 57개 과목에 걸친 객관식 문제 1만4000여 개의 집합인 MMLU 벤치마크를 아제르 번역을 이용한 다양한 언어로 번역했다(참조) 부록). 테스트된 26개 언어 중 24개 언어에서 GPT-4는 라트비아어, 웨일어, 스와힐리어와 같은 저자원 언어를 포함한 GPT-3.5와 다른 LLM(친칠라, PaLM)의 영어 성능을 능가한다.
비주얼 입력
GPT-4는 텍스트 및 이미지 프롬프트를 사용할 수 있으며, 이는 텍스트 전용 설정과 유사하게 사용자가 모든 비젼 또는 언어 작업을 지정할 수 있도록 합니다. 구체적으로, 인터퍼된 텍스트와 이미지로 구성된 입력이 주어진 텍스트 출력(자연어, 코드 등)을 생성한다. 텍스트 및 사진, 다이어그램 또는 스크린샷이 있는 문서를 포함한 다양한 도메인에서 GPT-4는 텍스트 전용 입력과 유사한 기능을 보여줍니다. 또한, 그것은 몇 번의 슛을 포함한 텍스트 전용 언어 모델을 위해 개발된 테스트 타임 기술로 증강될 수 있습니다. 연쇄적 사고 자극적이에요 이미지 입력은 여전히 연구 미리보기이며 공개적으로 사용할 수 없습니다.
영상 입력 : VGA 충전기
GPT-4는 일반적으로 대부분의 데이터가 절단된 후 발생한 사건에 대한 지식이 부족하고(2021년 9월)에서 배우지 못한다. 그것은 때때로 그렇게 많은 영역에 걸친 능력에 부합하지 않는 것처럼 보이는 단순한 추론 오류를 만들거나, 사용자의 명백한 거짓 진술을 받아들이는 데 지나치게 속을 수 있는 것으로 보인다. 그리고 때때로 그것은 인간이 만드는 코드에 보안 취약점을 도입하는 것과 같은 어려운 문제에 실패할 수 있습니다.
GPT-4는 또한 실수할 가능성이 있을 때 다시 한번 작업을 점검하는 것에 주의를 기울이지 않고 예측에서 자신 있게 틀릴 수 있다. 흥미롭게도, 베이스 미리 훈련된 모델은 높은 보정을 받는다(대답에 대한 예측된 신뢰는 일반적으로 정확할 확률과 일치한다). 그러나 현재 교육 후 과정을 통해 보정이 감소합니다.
챗GPT 플러스
ChatGPT Plus 가입자는 chat.openai.com 사용모가 있는 GPT-4 접속을 얻게 됩니다. openai.com 실제 수요와 시스템 성능에 따라 정확한 사용한도를 조정할 것이지만, 심각한 용량이 제한될 것으로 예상됩니다 (향후 몇 달 동안 규모를 확장하고 최적화할 것이지만).
우리가 보는 교통 패턴에 따라, 우리는 더 높은 볼륨의 GPT-4 사용에 대한 새로운 청약 수준을 도입할 수 있습니다; 또한 어느 시점에 구독이 없는 사람들도 시도할 수 있도록 일부 무료 GPT-4 쿼리를 제공하기를 희망합니다.
GPT-4
GPT-4 is more creative and collaborative than ever before. It can generate, edit, and iterate with users on creative and technical writing tasks, such as composing songs, writing screenplays, or learning a user’s writing style.
openai.com
API
Models
Overview
The OpenAI API is powered by a diverse set of models with different capabilities and price points. You can also make limited customizations to our original base models for your specific use case with fine-tuning.
GPT-4
Limited beta
|
A set of models that improve on GPT-3.5 and can understand as well as generate natural language or code |
GPT-3.5 | A set of models that improve on GPT-3 and can understand as well as generate natural language or code |
DALL·E
Beta
|
A model that can generate and edit images given a natural language prompt |
Whisper
Beta
|
A model that can convert audio into text |
Embeddings | A set of models that can convert text into a numerical form |
Codex
Limited beta
|
A set of models that can understand and generate code, including translating natural language to code |
Moderation | A fine-tuned model that can detect whether text may be sensitive or unsafe |
GPT-3 | A set of models that can understand and generate natural language |
We have also published open source models including Point-E, Whisper, Jukebox, and CLIP.
Visit our model index for researchers to learn more about which models have been featured in our research papers and the differences between model series like InstructGPT and GPT-3.5.
GPT-4
GPT-4 is a large multimodal model (accepting text inputs and emitting text outputs today, with image inputs coming in the future) that can solve difficult problems with greater accuracy than any of our previous models, thanks to its broader general knowledge and advanced reasoning capabilities. Like gpt-3.5-turbo, GPT-4 is optimized for chat but works well for traditional completions tasks. Learn how to use GPT-4 in our chat guide.
gpt-4 | More capable than any GPT-3.5 model, able to do more complex tasks, and optimized for chat. Will be updated with our latest model iteration. | 8,192 tokens | Up to Sep 2021 |
gpt-4-0314 | Snapshot of gpt-4 from March 14th 2023. Unlike gpt-4, this model will not receive updates, and will only be supported for a three month period ending on June 14th 2023. | 8,192 tokens | Up to Sep 2021 |
gpt-4-32k | Same capabilities as the base gpt-4 mode but with 4x the context length. Will be updated with our latest model iteration. | 32,768 tokens | Up to Sep 2021 |
gpt-4-32k-0314 | Snapshot of gpt-4-32 from March 14th 2023. Unlike gpt-4-32k, this model will not receive updates, and will only be supported for a three month period ending on June 14th 2023. | 32,768 tokens | Up to Sep 2021 |
For many basic tasks, the difference between GPT-4 and GPT-3.5 models is not significant. However, in more complex reasoning situations, GPT-4 is much more capable than any of our previous models.
GPT-3.5
GPT-3.5 models can understand and generate natural language or code. Our most capable and cost effective model in the GPT-3.5 family is gpt-3.5-turbo which has been optimized for chat but works well for traditional completions tasks as well.
gpt-3.5-turbo | Most capable GPT-3.5 model and optimized for chat at 1/10th the cost of text-davinci-003. Will be updated with our latest model iteration. | 4,096 tokens | Up to Sep 2021 |
gpt-3.5-turbo-0301 | Snapshot of gpt-3.5-turbo from March 1st 2023. Unlike gpt-3.5-turbo, this model will not receive updates, and will only be supported for a three month period ending on June 1st 2023. | 4,096 tokens | Up to Sep 2021 |
text-davinci-003 | Can do any language task with better quality, longer output, and consistent instruction-following than the curie, babbage, or ada models. Also supports inserting completions within text. | 4,097 tokens | Up to Jun 2021 |
text-davinci-002 | Similar capabilities to text-davinci-003 but trained with supervised fine-tuning instead of reinforcement learning | 4,097 tokens | Up to Jun 2021 |
code-davinci-002 | Optimized for code-completion tasks | 8,001 tokens | Up to Jun 2021 |
We recommend using gpt-3.5-turbo over the other GPT-3.5 models because of its lower cost.
Feature-specific models
While the new gpt-3.5-turbo model is optimized for chat, it works very well for traditional completion tasks. The original GPT-3.5 models are optimized for text completion.
Our endpoints for creating embeddings and editing text use their own sets of specialized models.
Finding the right model
Experimenting with gpt-3.5-turbo is a great way to find out what the API is capable of doing. After you have an idea of what you want to accomplish, you can stay with gpt-3.5-turbo or another model and try to optimize around its capabilities.
You can use the GPT comparison tool that lets you run different models side-by-side to compare outputs, settings, and response times and then download the data into an Excel spreadsheet.
DALL·E
DALL·E is a AI system that can create realistic images and art from a description in natural language. We currently support the ability, given a prommpt, to create a new image with a certain size, edit an existing image, or create variations of a user provided image.
The current DALL·E model available through our API is the 2nd iteration of DALL·E with more realistic, accurate, and 4x greater resolution images than the original model. You can try it through the our Labs interface or via the API.
Whisper
Whisper is a general-purpose speech recognition model. It is trained on a large dataset of diverse audio and is also a multi-task model that can perform multilingual speech recognition as well as speech translation and language identification. The Whisper v2-large model is currently available through our API with the whisper-1 model name.
Currently, there is no difference between the open source version of Whisper and the version available through our API. However, through our API, we offer an optimized inference process which makes running Whisper through our API much faster than doing it through other means. For more technical details on Whisper, you can read the paper.
Embeddings
Embeddings are a numerical representation of text that can be used to measure the relateness between two pieces of text. Our second generation embedding model, text-embedding-ada-002 is a designed to replace the previous 16 first-generation embedding models at a fraction of the cost. Embeddings are useful for search, clustering, recommendations, anomaly detection, and classification tasks. You can read more about our latest embedding model in the announcement blog post.
Codex
The Codex models are descendants of our GPT-3 models that can understand and generate code. Their training data contains both natural language and billions of lines of public code from GitHub. Learn more.
They’re most capable in Python and proficient in over a dozen languages including JavaScript, Go, Perl, PHP, Ruby, Swift, TypeScript, SQL, and even Shell.
We currently offer two Codex models:
code-davinci-002 | Most capable Codex model. Particularly good at translating natural language to code. In addition to completing code, also supports inserting completions within code. | 8,001 tokens | Up to Jun 2021 |
code-cushman-001 | Almost as capable as Davinci Codex, but slightly faster. This speed advantage may make it preferable for real-time applications. | Up to 2,048 tokens |
For more, visit our guide on working with Codex.
The Codex models are free to use during the limited beta, and are subject to reduced rate limits. As we learn about use, we'll look to offer pricing to enable a broad set of applications.
During this period, you're welcome to go live with your application as long as it follows our usage policies. We welcome any feedback on these models while in early use and look forward to engaging with the community.
Feature-specific models
The main Codex models are meant to be used with the text completion endpoint. We also offer models that are specifically meant to be used with our endpoints for creating embeddings and editing code.
Moderation
The Moderation models are designed to check whether content complies with OpenAI's usage policies. The models provide classification capabilities that look for content in the following categories: hate, hate/threatening, self-harm, sexual, sexual/minors, violence, and violence/graphic. You can find out more in our moderation guide.
Moderation models take in an arbitrary sized input that is automatically broken up to fix the models specific context window.
MODELDESCRIPTIONtext-moderation-latest | Most capable moderation model. Accuracy will be slighlty higher than the stable model |
text-moderation-stable | Almost as capable as the latest model, but slightly older. |
GPT-3
GPT-3 models can understand and generate natural language. These models were superceded by the more powerful GPT-3.5 generation models. However, the original GPT-3 base models (davinci, curie, ada, and babbage) are current the only models that are available to fine-tune.
text-curie-001 | Very capable, faster and lower cost than Davinci. | 2,049 tokens | Up to Oct 2019 |
text-babbage-001 | Capable of straightforward tasks, very fast, and lower cost. | 2,049 tokens | Up to Oct 2019 |
text-ada-001 | Capable of very simple tasks, usually the fastest model in the GPT-3 series, and lowest cost. | 2,049 tokens | Up to Oct 2019 |
davinci | Most capable GPT-3 model. Can do any task the other models can do, often with higher quality. | 2,049 tokens | Up to Oct 2019 |
curie | Very capable, but faster and lower cost than Davinci. | 2,049 tokens | Up to Oct 2019 |
babbage | Capable of straightforward tasks, very fast, and lower cost. | 2,049 tokens | Up to Oct 2019 |
ada | Capable of very simple tasks, usually the fastest model in the GPT-3 series, and lowest cost. | 2,049 tokens | Up to Oct 2019 |
Model endpoint compatibility
/v1/chat/completions | gpt-4, gpt-4-0314, gpt-4-32k, gpt-4-32k-0314, gpt-3.5-turbo, gpt-3.5-turbo-0301 | |
/v1/completions | text-davinci-003, text-davinci-002, text-curie-001, text-babbage-001, text-ada-001, davinci, curie, babbage, ada | |
/v1/edits text-davinci-edit-001 | text-davinci-edit-001, code-davinci-edit-001 | |
/v1/audio/transcriptions | whisper-1 | |
/v1/audio/translations | whisper-1 | |
/v1/fine-tunes | davinci, curie, babbage, ada | |
/v1/embeddings | text-embedding-ada-002, text-search-ada-doc-001 | |
/v1/moderations | text-moderation-stable, text-moderation-latest |
This list does not include our first-generation embedding models nor our DALL·E models.
Continuous model upgrades
With the release of gpt-3.5-turbo, some of our models are now being continually updated. In order to mitigate the chance of model changes affecting our users in an unexpected way, we also offer model versions that will stay static for 3 month periods. With the new cadence of model updates, we are also giving people the ability to contribute evals to help us improve the model for different use cases. If you are interested, check out the OpenAI Evals repository.
The following models are the temporary snapshots that will be deprecated at the specified date. If you want to use the latest model version, use the standard model names like gpt-4 or gpt-3.5-turbo.
MODEL NAMEDEPRECATION DATEgpt-3.5-turbo-0301 | June 1st, 2023 | |
gpt-4-0314 | June 14th, 2023 | |
gpt-4-32k-0314 | June 14th, 2023 |
|
ChatGPT plugins
We’ve implemented initial support for plugins in ChatGPT. Plugins are tools designed specifically for language models with safety as a core principle, and help ChatGPT access up-to-date information, run computations, or use third-party services.
Quick links
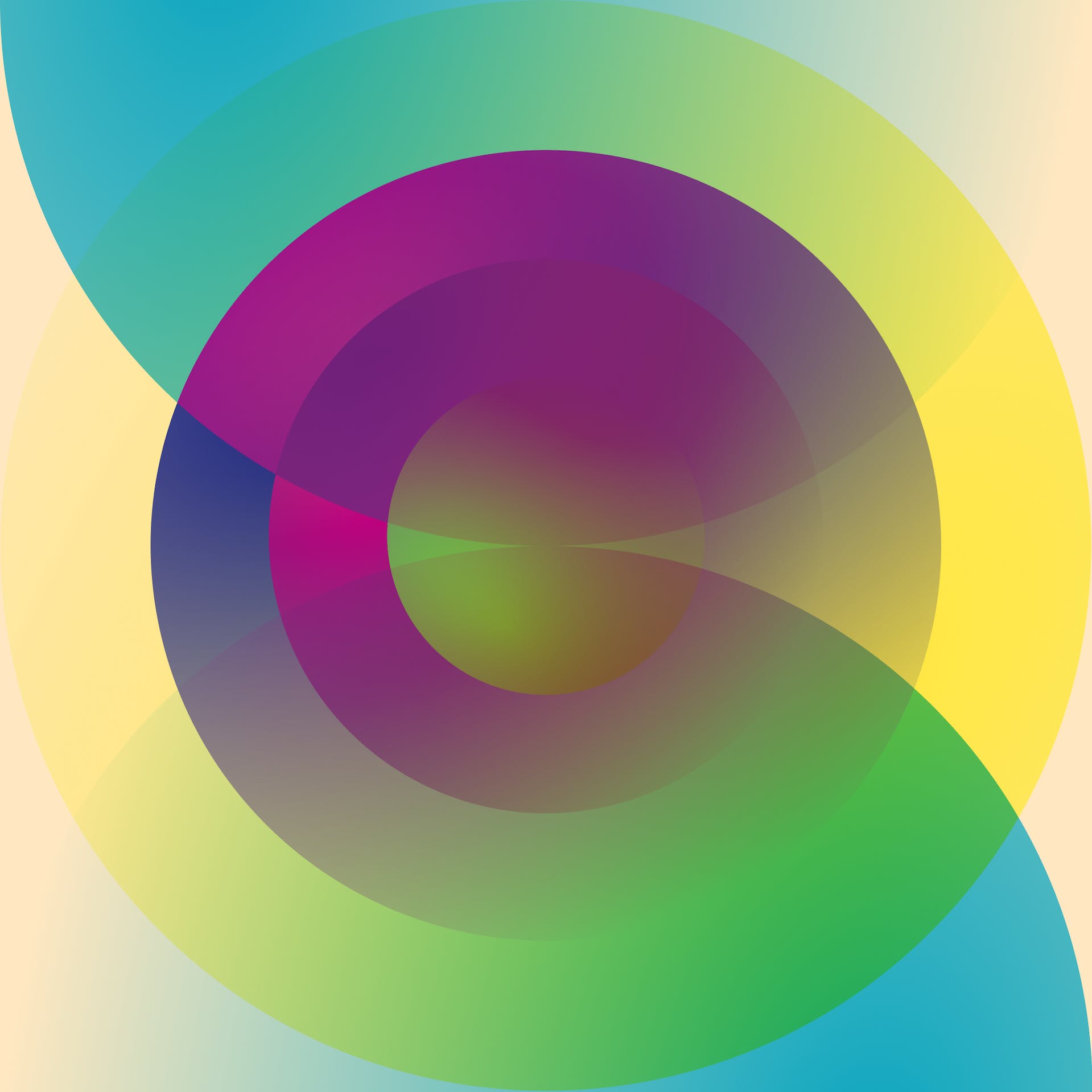
Ruby Chen
In line with our iterative deployment philosophy, we are gradually rolling out plugins in ChatGPT so we can study their real-world use, impact, and safety and alignment challenges—all of which we’ll have to get right in order to achieve our mission.
Users have been asking for plugins since we launched ChatGPT (and many developers are experimenting with similar ideas) because they unlock a vast range of possible use cases. We’re starting with a small set of users and are planning to gradually roll out larger-scale access as we learn more (for plugin developers, ChatGPT users, and after an alpha period, API users who would like to integrate plugins into their products). We’re excited to build a community shaping the future of the human–AI interaction paradigm.
Plugin developers who have been invited off our waitlist can use our documentation to build a plugin for ChatGPT, which then lists the enabled plugins in the prompt shown to the language model as well as documentation to instruct the model how to use each. The first plugins have been created by Expedia, FiscalNote, Instacart, KAYAK, Klarna, Milo, OpenTable, Shopify, Slack, Speak, Wolfram, and Zapier.
We’re also hosting two plugins ourselves, a web browser and code interpreter. We’ve also open-sourced the code for a knowledge base retrieval plugin, to be self-hosted by any developer with information with which they’d like to augment ChatGPT.
Today, we will begin extending plugin alpha access to users and developers from our waitlist. While we will initially prioritize a small number of developers and ChatGPT Plus users, we plan to roll out larger-scale access over time.
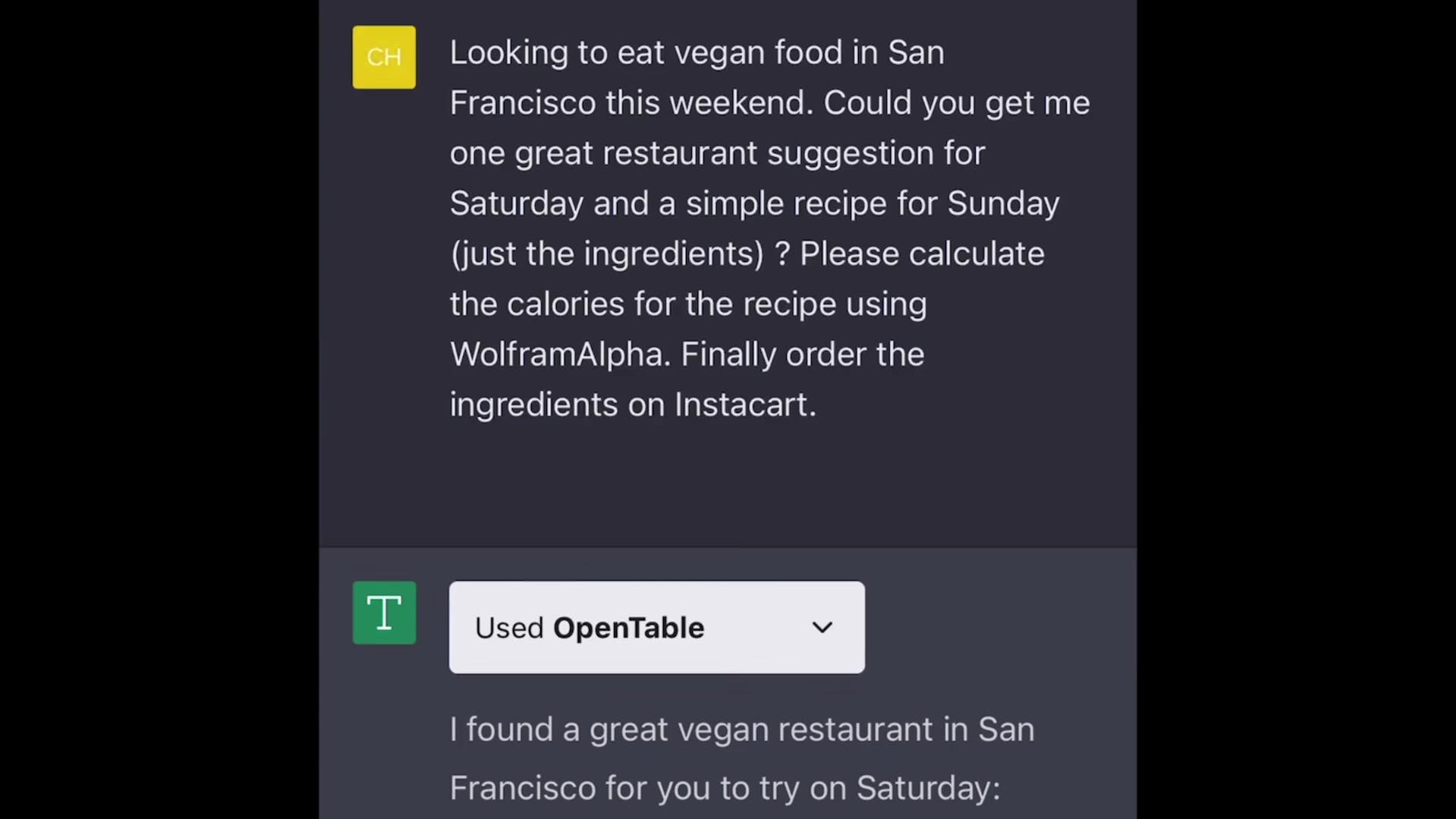
Overview
Language models today, while useful for a variety of tasks, are still limited. The only information they can learn from is their training data. This information can be out-of-date and is one-size fits all across applications. Furthermore, the only thing language models can do out-of-the-box is emit text. This text can contain useful instructions, but to actually follow these instructions you need another process.
Though not a perfect analogy, plugins can be “eyes and ears” for language models, giving them access to information that is too recent, too personal, or too specific to be included in the training data. In response to a user’s explicit request, plugins can also enable language models to perform safe, constrained actions on their behalf, increasing the usefulness of the system overall.
We expect that open standards will emerge to unify the ways in which applications expose an AI-facing interface. We are working on an early attempt at what such a standard might look like, and we’re looking for feedback from developers interested in building with us.
Today, we’re beginning to gradually enable existing plugins from our early collaborators for ChatGPT users, beginning with ChatGPT Plus subscribers. We’re also beginning to roll out the ability for developers to create their own plugins for ChatGPT.
In the coming months, as we learn from deployment and continue to improve our safety systems, we’ll iterate on this protocol, and we plan to enable developers using OpenAI models to integrate plugins into their own applications beyond ChatGPT.
Safety and broader implications
Connecting language models to external tools introduces new opportunities as well as significant new risks.
Plugins offer the potential to tackle various challenges associated with large language models, including “hallucinations,” keeping up with recent events, and accessing (with permission) proprietary information sources. By integrating explicit access to external data—such as up-to-date information online, code-based calculations, or custom plugin-retrieved information—language models can strengthen their responses with evidence-based references.
These references not only enhance the model’s utility but also enable users to assess the trustworthiness of the model’s output and double-check its accuracy, potentially mitigating risks related to overreliance as discussed in our recent GPT-4 system card. Lastly, the value of plugins may go well beyond addressing existing limitations by helping users with a variety of new use cases, ranging from browsing product catalogs to booking flights or ordering food.
At the same time, there’s a risk that plugins could increase safety challenges by taking harmful or unintended actions, increasing the capabilities of bad actors who would defraud, mislead, or abuse others. By increasing the range of possible applications, plugins may raise the risk of negative consequences from mistaken or misaligned actions taken by the model in new domains. From day one, these factors have guided the development of our plugin platform, and we have implemented several safeguards.
From day one, these factors have guided the development of our plugin platform, and we have implemented several safeguards.
We’ve performed red-teaming exercises, both internally and with external collaborators, that have revealed a number of possible concerning scenarios. For example, our red teamers discovered ways for plugins—if released without safeguards—to perform sophisticated prompt injection, send fraudulent and spam emails, bypass safety restrictions, or misuse information sent to the plugin. We’re using these findings to inform safety-by-design mitigations that restrict risky plugin behaviors and improve transparency of how and when they're operating as part of the user experience. We're also using these findings to inform our decision to gradually deploy access to plugins.
If you’re a researcher interested in studying safety risks or mitigations in this area, we encourage you to make use of our Researcher Access Program. We also invite developers and researchers to submit plugin-related safety and capability evaluations as part of our recently open-sourced Evals framework.
Plugins will likely have wide-ranging societal implications. For example, we recently released a working paper which found that language models with access to tools will likely have much greater economic impacts than those without, and more generally, in line with other researchers’ findings, we expect the current wave of AI technologies to have a big effect on the pace of job transformation, displacement, and creation. We are eager to collaborate with external researchers and our customers to study these impacts.
Browsing
AlphaMotivated by past work (our own WebGPT, as well as GopherCite, BlenderBot2, LaMDA2 and others), allowing language models to read information from the internet strictly expands the amount of content they can discuss, going beyond the training corpus to fresh information from the present day.
Here’s an example of the kind of experience that browsing opens up to ChatGPT users, that previously would have had the model politely point out that its training data didn’t include enough information to let it answer. This example, in which ChatGPT retrieves recent information about the latest Oscars, and then performs now-familiar ChatGPT poetry feats, is one way that browsing can be an additive experience.
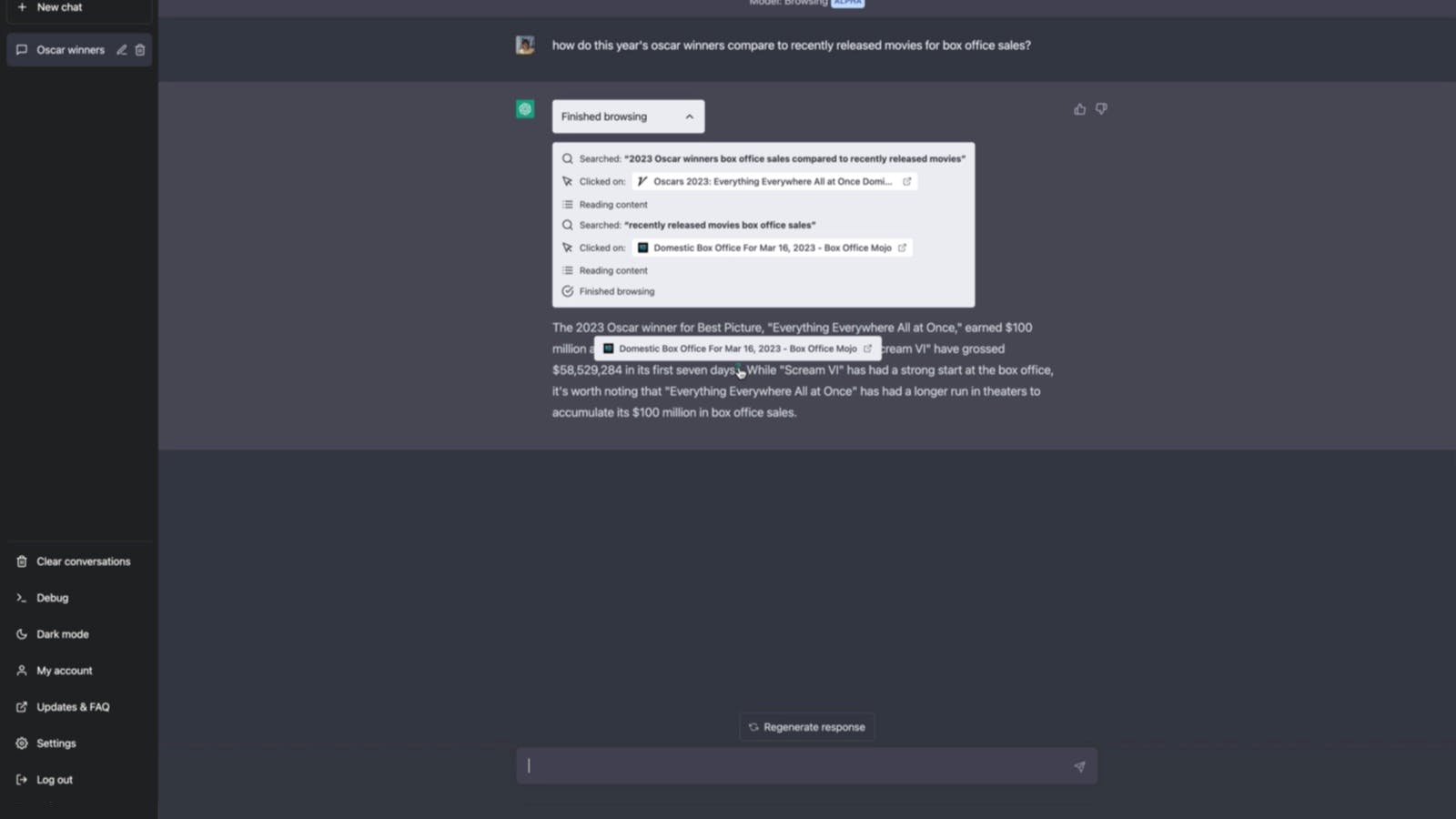
In addition to providing obvious utility to end-users, we think enabling language and chat models to do thorough and interpretable research has exciting prospects for scalable alignment.
Safety considerations
We’ve created a web browsing plugin which gives a language model access to a web browser, with its design prioritizing both safety and operating as a good citizen of the web. The plugin’s text-based web browser is limited to making GET requests, which reduces (but does not eliminate) certain classes of safety risks. This scopes the browsing plugin to be useful for retrieving information, but excludes “transactional” operations such as form submission which have more surface area for security and safety issues.
Browsing retrieves content from the web using the Bing search API. As a result, we inherit substantial work from Microsoft on (1) source reliability and truthfulness of information and (2) “safe-mode” to prevent the retrieval of problematic content. The plugin operates within an isolated service, so ChatGPT’s browsing activities are separated from the rest of our infrastructure.
To respect content creators and adhere to the web’s norms, our browser plugin’s user-agent token is ChatGPT-User and is configured to honor websites' robots.txt files. This may occasionally result in a “click failed” message, which indicates that the plugin is honoring the website's instruction to avoid crawling it. This user-agent will only be used to take direct actions on behalf of ChatGPT users and is not used for crawling the web in any automatic fashion. We have also published our IP egress ranges. Additionally, rate-limiting measures have been implemented to avoid sending excessive traffic to websites.
Our browsing plugin shows websites visited and cites its sources in ChatGPT’s responses. This added layer of transparency helps users verify the accuracy of the model’s responses and also gives credit back to content creators. We appreciate that this is a new method of interacting with the web, and welcome feedback on additional ways to drive traffic back to sources and add to the overall health of the ecosystem.
Code interpreter
AlphaWe provide our models with a working Python interpreter in a sandboxed, firewalled execution environment, along with some ephemeral disk space. Code run by our interpreter plugin is evaluated in a persistent session that is alive for the duration of a chat conversation (with an upper-bound timeout) and subsequent calls can build on top of each other. We support uploading files to the current conversation workspace and downloading the results of your work.
We would like our models to be able to use their programming skills to provide a much more natural interface to most fundamental capabilities of our computers. Having access to a very eager junior programmer working at the speed of your fingertips can make completely new workflows effortless and efficient, as well as open the benefits of programming to new audiences.
From our initial user studies, we’ve identified use cases where using code interpreter is especially useful:
- Solving mathematical problems, both quantitative and qualitative
- Doing data analysis and visualization
- Converting files between formats
We invite users to try the code interpreter integration and discover other useful tasks.
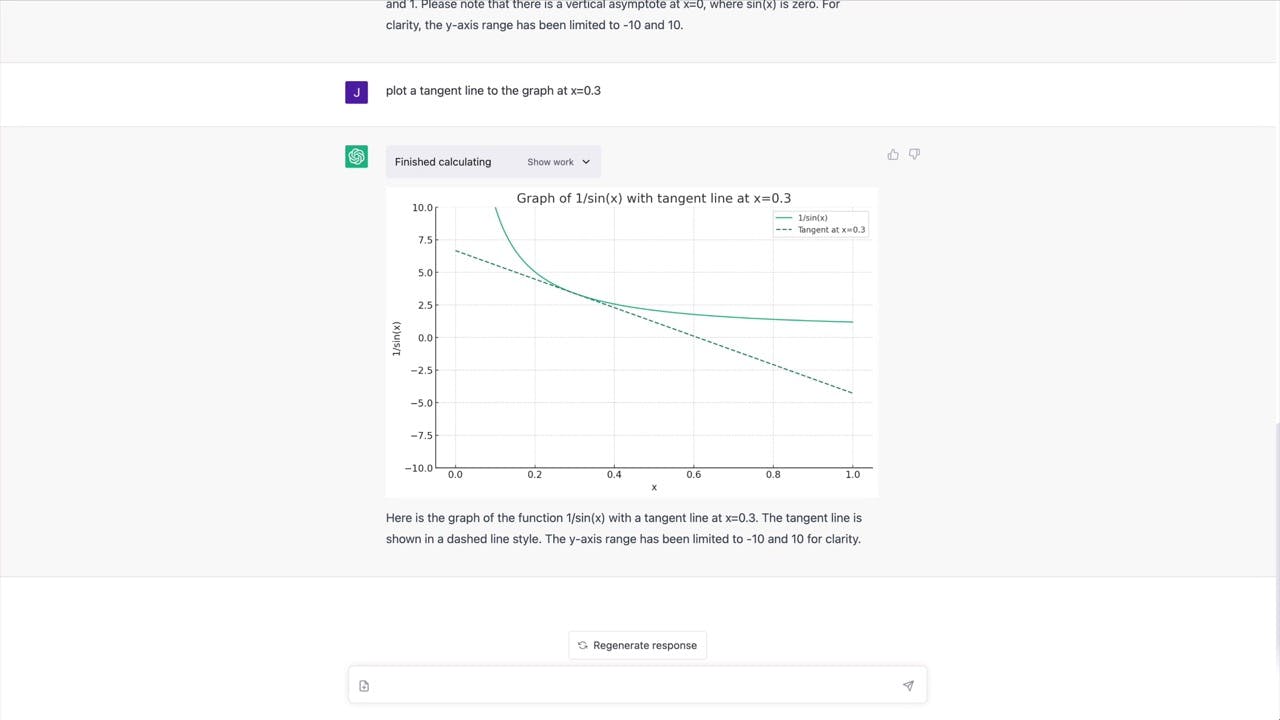
Safety considerations
The primary consideration for connecting our models to a programming language interpreter is properly sandboxing the execution so that AI-generated code does not have unintended side-effects in the real world. We execute code in a secured environment and use strict network controls to prevent external internet access from executed code. Additionally, we have set resource limits on each session. Disabling internet access limits the functionality of our code sandbox, but we believe it’s the right initial tradeoff. Third-party plugins were designed as a safety-first method of connecting our models to the outside world.
Retrieval
The open-source retrieval plugin enables ChatGPT to access personal or organizational information sources (with permission). It allows users to obtain the most relevant document snippets from their data sources, such as files, notes, emails or public documentation, by asking questions or expressing needs in natural language.
As an open-source and self-hosted solution, developers can deploy their own version of the plugin and register it with ChatGPT. The plugin leverages OpenAI embeddings and allows developers to choose a vector database (Milvus, Pinecone, Qdrant, Redis, Weaviate or Zilliz) for indexing and searching documents. Information sources can be synchronized with the database using webhooks.
To begin, visit the retrieval plugin repository.
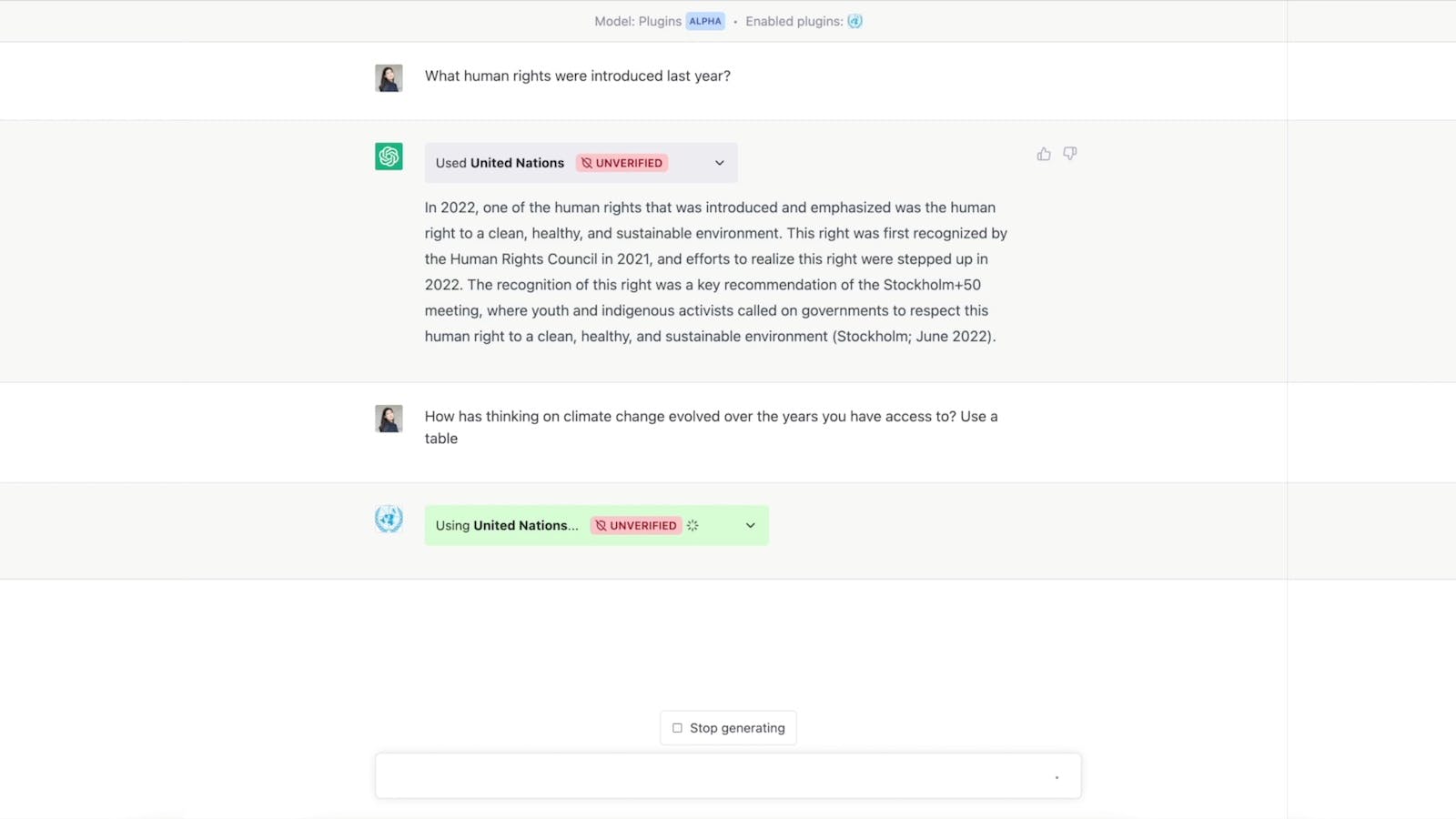
Security considerations
The retrieval plugin allows ChatGPT to search a vector database of content, and add the best results into the ChatGPT session. This means it doesn’t have any external effects, and the main risk is data authorization and privacy. Developers should only add content into their retrieval plugin that they are authorized to use and can share in users’ ChatGPT sessions.
Third-party plugins
AlphaThird-party plugins are described by a manifest file, which includes a machine-readable description of the plugin’s capabilities and how to invoke them, as well as user-facing documentation.
{
"schema_version": "v1",
"name_for_human": "TODO Manager",
"name_for_model": "todo_manager",
"description_for_human": "Manages your TODOs!",
"description_for_model": "An app for managing a user's TODOs",
"api": { "url": "/openapi.json" },
"auth": { "type": "none" },
"logo_url": "https://example.com/logo.png",
"legal_info_url": "http://example.com",
"contact_email": "hello@example.com"
}
An example manifest file for a plugin for managing to-dos
The steps for creating a plugin are:
- Build an API with endpoints you’d like a language model to call (this can be a new API, an existing API, or a wrapper around an existing API specifically designed for LLMs).
- Create an OpenAPI specification documenting your API, and a manifest file that links to the OpenAPI spec and includes some plugin-specific metadata.
When starting a conversation on chat.openai.com, users can choose which third-party plugins they’d like to be enabled. Documentation about the enabled plugins is shown to the language model as part of the conversation context, enabling the model to invoke appropriate plugin APIs as needed to fulfill user intent. For now, plugins are designed for calling backend APIs, but we are exploring plugins that can call client-side APIs as well.
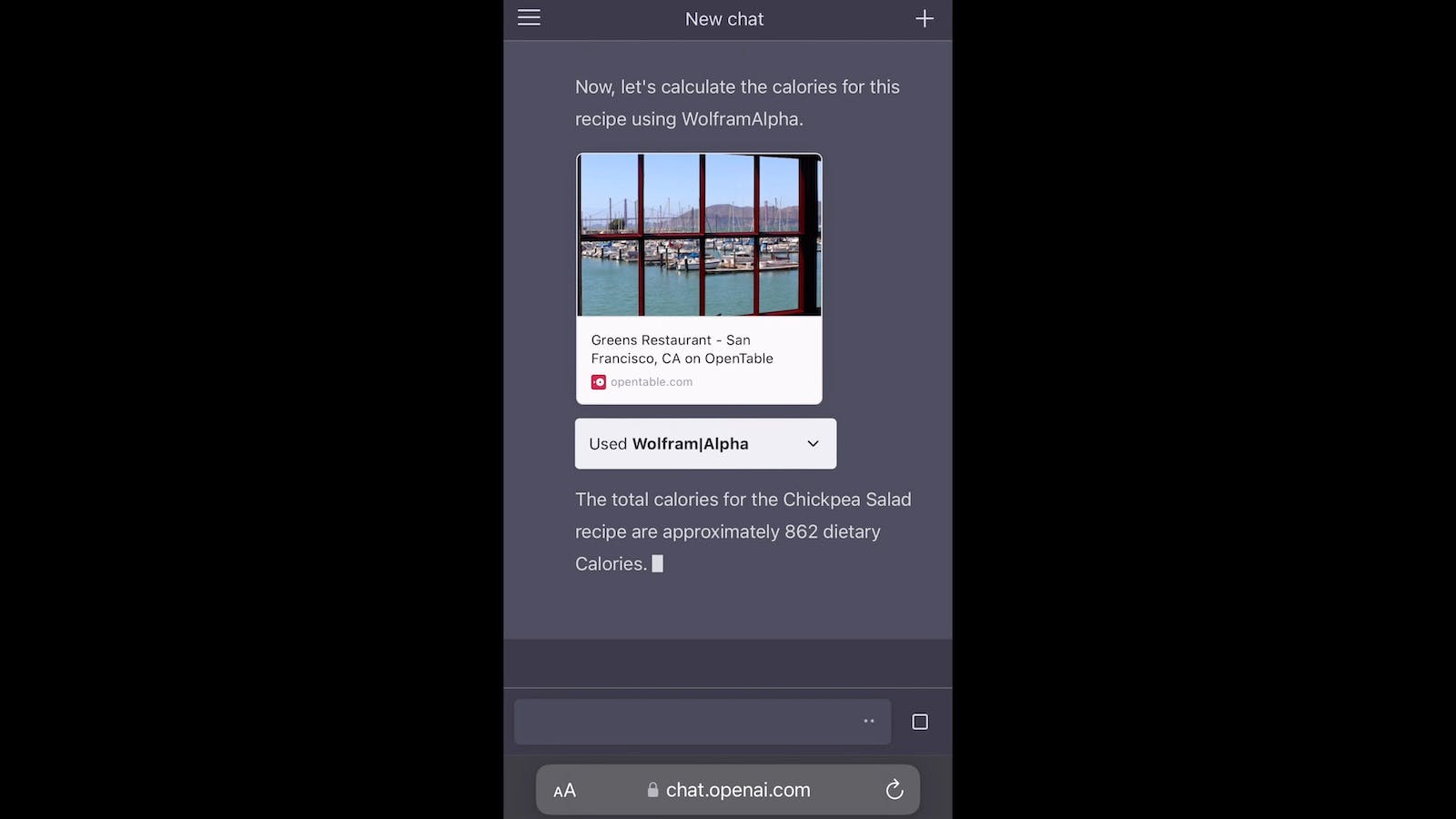
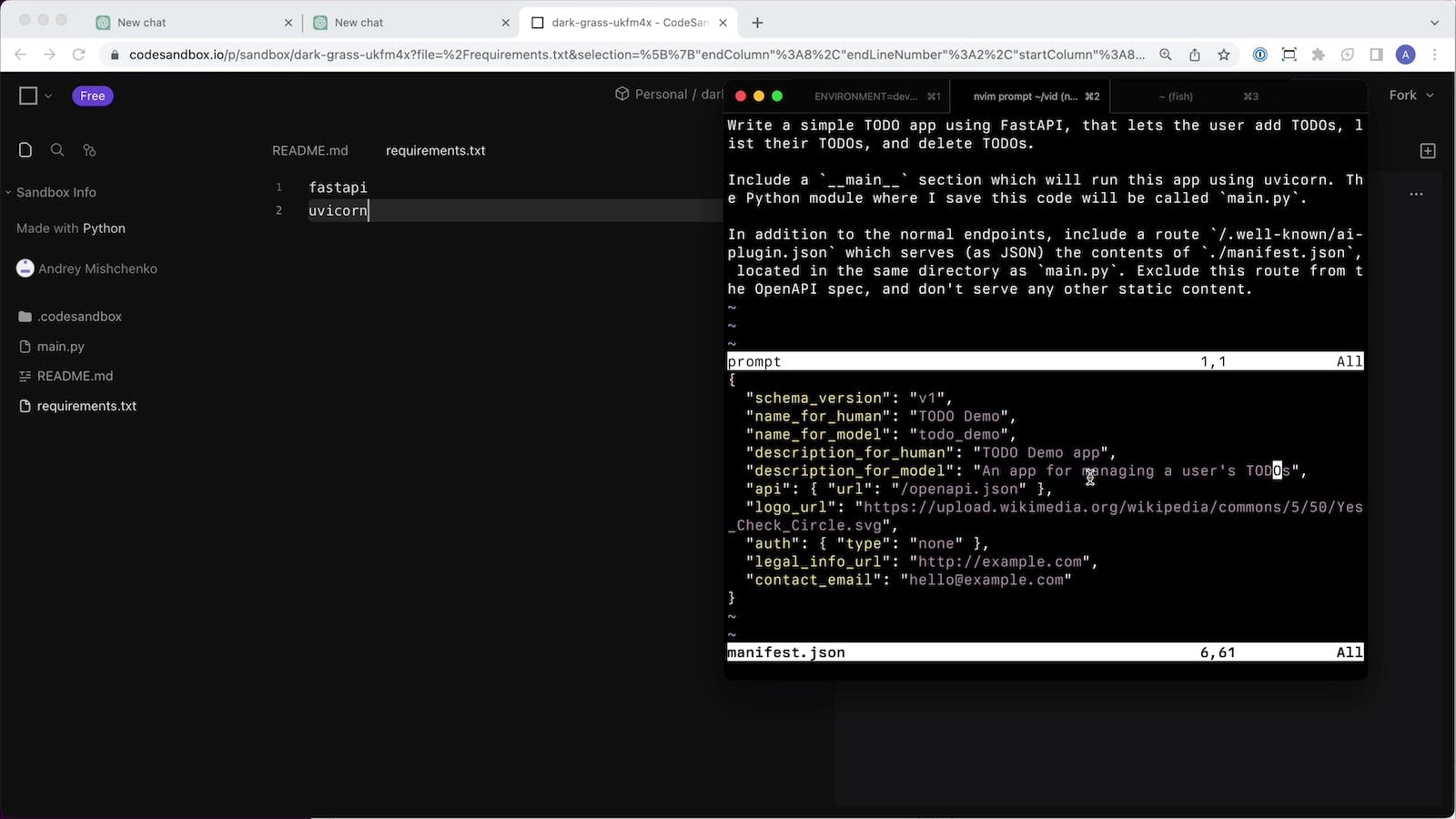
Looking forward
We’re working to develop plugins and bring them to a broader audience. We have a lot to learn, and with the help of everyone, we hope to build something that is both useful and safe.
Authors
-
OpenAI
View all articles
Acknowledgments
Contributors
Sandhini Agarwal, Ilge Akkaya, Valerie Balcom, Mo Bavarian, Gabriel Bernadett-Shapiro, Greg Brockman, Miles Brundage, Jeff Chan, Fotis Chantzis, Noah Deutsch, Brydon Eastman, Atty Eleti, Niko Felix, Simón Posada Fishman, Isa Fulford, Christian Gibson, Joshua Gross, Mike Heaton, Jacob Hilton, Xin Hu, Shawn Jain, Haozhun Jin, Logan Kilpatrick, Christina Kim, Michael Kolhede, Andrew Mayne, Paul McMillan, David Medina, Jacob Menick, Andrey Mishchenko, Ashvin Nair, Rajeev Nayak, Arvind Neelakantan, Rohan Nuttall, Joel Parish, Alex Tachard Passos, Adam Perelman, Filipe de Avila Belbute Peres, Vitchyr Pong, John Schulman, Eric Sigler, Natalie Staudacher, Nicholas Turley, Jerry Tworek, Ryan Greene, Arun Vijayvergiya, Chelsea Voss, Jiayi Weng, Matt Wiethoff, Sarah Yoo, Kevin Yu, Wojciech Zaremba, Shengjia Zhao, Will Zhuk, Barret Zoph
Related research
-
GPTs are GPTs: An early look at the labor market impact potential of large language models
March 17, 2023 -
GPT-4
March 14, 2023 -
Forecasting potential misuses of language models for disinformation campaigns and how to reduce risk
January 11, 2023 -
Point-E: A system for generating 3D point clouds from complex prompts
December 16, 2022
Safety
구글의 반격
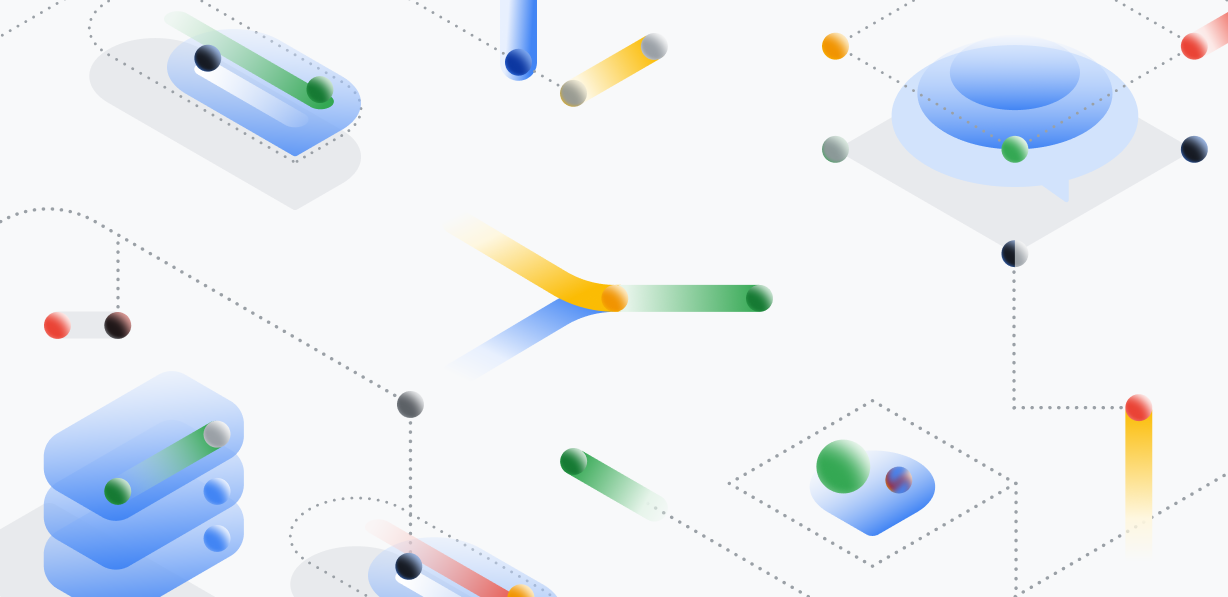
PaLM API & MakerSuite: an approachable way to start prototyping and building generative AI applications
March 14, 2023
Posted by Scott Huffman, Vice President, Engineering and Josh Woodward, Senior Director, Product Management
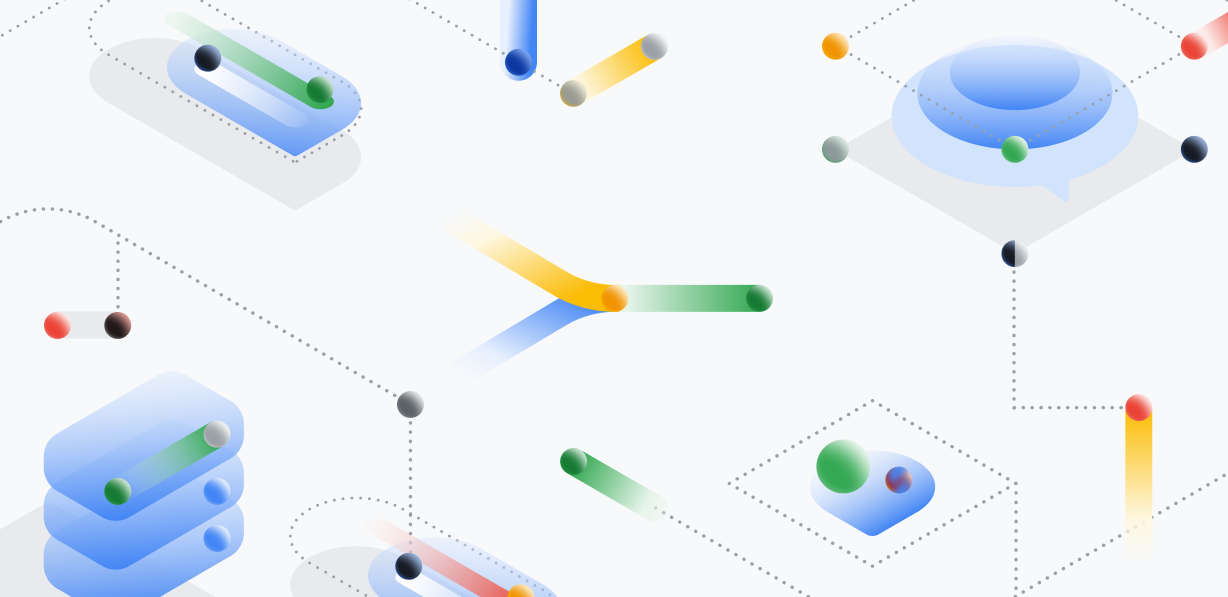
We’re seeing a new wave of generative AI applications that are transforming the way people interact with technology – from games and dialog agents to creative brainstorming and coding tools. At Google, we want to continue making AI accessible by empowering all developers to start building the next generation of applications with generative AI by providing easy-to-use APIs and tools.
Earlier today, we announced the PaLM API, a new developer offering that makes it easy and safe to experiment with Google’s large language models. Alongside the API, we’re releasing MakerSuite, a tool that lets developers start prototyping quickly and easily. We’ll be making these tools available to select developers through a Private Preview, and stay tuned for our waitlist soon.
Access Google’s large language models using the PaLM APIThe PaLM API is a simple entry point for Google’s large language models, which can be used for a variety of applications. It will provide developers access to models that are optimized for multi-turn use cases, such as content generation and chat, and general purpose models that are optimized for use cases such as summarization, classification, and more. Starting today, we’re making an efficient model available in terms of size and capabilities, and we’ll add other models and sizes soon. |
|
Start building quicklyWe’ve spent the last several years building and deploying large language models—from bringing MUM to Search to exploring applications with LaMDA in the AI Test Kitchen. We learned a lot about generative AI development workflows and how fragmented they can be. Developers have to use different tools to accomplish tasks like crafting and iterating on a prompt, generating synthetic data, and tuning a custom model.That’s why we’re releasing MakerSuite, a tool that simplifies this workflow. With MakerSuite, you’ll be able to iterate on prompts, augment your dataset with synthetic data, and easily tune custom models. When you’re ready to move to code, MakerSuite will let you export your prompt as code in your favorite languages and frameworks, like Python and Node.js. |
|
Tune a modelGenerative models offer developers powerful out-of-the-box functionality. But for specialized tasks, tuning leads to better results. Our tooling will enable developers to leverage parameter-efficient tuning techniques to create models customized to their use case. And with MakerSuite, you’ll be able to quickly test and iterate on your tuned model right in the browser. |
|
Augment your dataset with synthetic dataHigh-quality data is crucial when developing with AI, and developers are often limited by the data they have. Our tooling will allow you to generate additional data based on a few examples, and then you’ll be able to manage and manipulate the data from there. This synthetic data can be used in various scenarios, such as tuning or evaluations. |
|
Generate state of the art embeddingsWe’ve been excited by the range of applications developers have found for embeddings, from semantic search to recommendations and classification. With embeddings generated through the PaLM API, developers will be able to build applications with their own data or on top of external data sources. Embeddings can also be used in downstream applications built with TensorFlow, Keras, JAX, and other open-source libraries. |
|
Build responsibly and safelyWe built our models according to Google’s AI Principles to give developers a responsible AI foundation to start from. We know that control is necessary so developers can define and enforce responsibility and safety in the context of their own applications. Our tools will give developers an easy way to test and adjust safety dimensions to best suit each unique application and use case. |
|
Scale your generative AI applicationThese developer tools will make it easy to start prototyping and building generative AI applications, but when you need scale, we want to make sure you have the support you need. Google's infrastructure supports the PaLM API and MakerSuite, so you don’t have to worry about hosting or serving. For developers who want to scale their ideas and get enterprise-grade support, security and compliance, and service level agreement (SLA), they can go to Google Cloud Vertex AI and access the same models, along with a host of advanced capabilities such as enterprise search and conversation AI. |
It’s an exciting time in AI for developers and we want to continue to make sure we build AI tools that help make your lives easier. We plan to onboard new developers, roll out new features, and make this technology available to the broader developer community soon. During this time, we’ll listen to feedback, learn, and improve these tools to meet developers where they are.
To stay updated on our progress, subscribe to the Google Developers newsletter.